Project – Analysing Host-Microbiome Interactions
The microbiome plays a vital role in maintaining host systems' homeostatic (healthy) functions. Disrupting the healthy microbiota - by external (temperature, hygiene, etc.) or internal factors (inflammation, ageing, etc.) - causes a dysbiotic (unhealthy) state. Disruption of the microbial equilibrium between commensal and pathogen species could lead to the development of various disorders. Though differences in the microbiota between healthy and unhealthy phenotypes have been reported, the mechanistic role of the microbiota in modulating homeostatic processes remains largely unexplored.
As a focused, gap-filling attempt to address this problem, we have developed in silico pipelines to predict host-microbe interactions and analyse the effect of microbes on human signalling pathways based on omics data using network biology approaches. In industrial collaborations with BenevolentAI and Unilever, we have been testing and fine-tuning our workflows on data from the gastrointestinal tract.
The gut microbiome primes the immune system during early development and contributes to lifelong homeostasis. Several disorders, such as dementia and Inflammatory Bowel Disease (IBD), are associated with gut microbiome alterations. Despite developments in generating microbiome data, the critical obstacle remains - which is to extract predictive biomarkers. This problem consists of the processing of these large datasets and the fact that microbiome studies are often correlative and therefore lack the mechanism of host-microbiota interactions. Furthermore, there are many unknown microbes (mostly commensals, unicellular eukaryotes, viruses) whose health-contributing potential continues to be unclear. This, in turn, means these datasets remain very noisy and incomplete, thus requiring complex analytical methods.
To address these gaps, we have built up an integrated machine learning-based systems biology pipeline in industrial cooperation with BenevolentAI. The pipeline is applied to the gut microbiome data for identifying prognostic indicators of healthy ageing and age-related disorders. The approach is based on a multi-omic approach, metagenomics, metatranscriptomics and metabolomic data, which captures the composition and functional potential of the microbiome in modulating host processes.
We also aimed to develop bioinformatic pipelines, such as MicrobioLink [Figure 1] and ViralLink [Figure 2], to predict host-microbe interactions and analyse how the host responds to the interkingdom interactions. We use not only bulk transcriptomics - which describes gene expression on tissue or cell type level - but we also developed our scOmix pipeline to process and examine single-cell transcriptomics data to highlight cell-type-specific gene expression patterns. We collaborated with Unilever to apply these analyses on transcriptomic data on samples derived from healthy and inflamed gingiva.
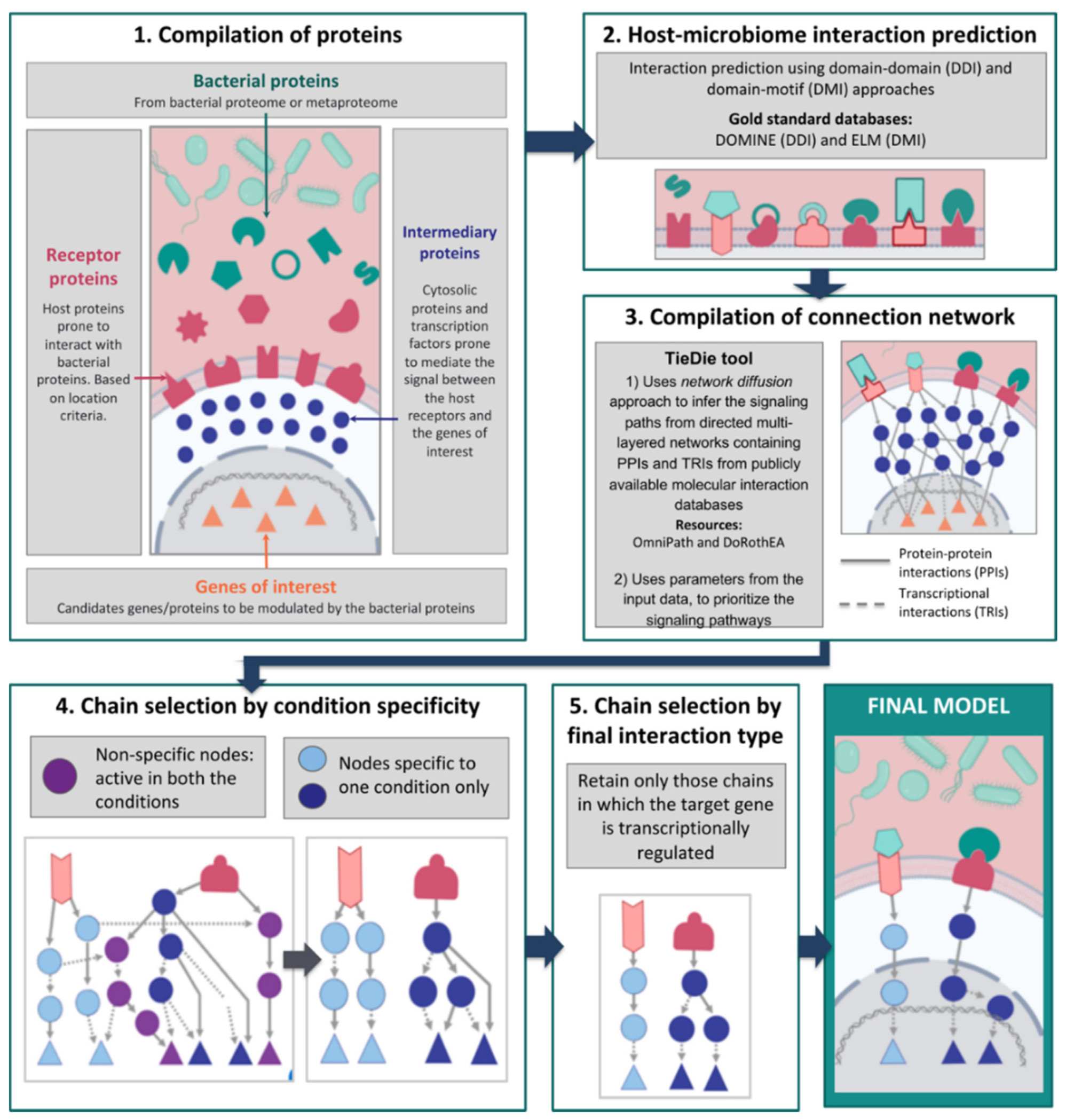
Figure 1: Workflow of the MicrobioLink tool
For full-sized version, please click on the image.
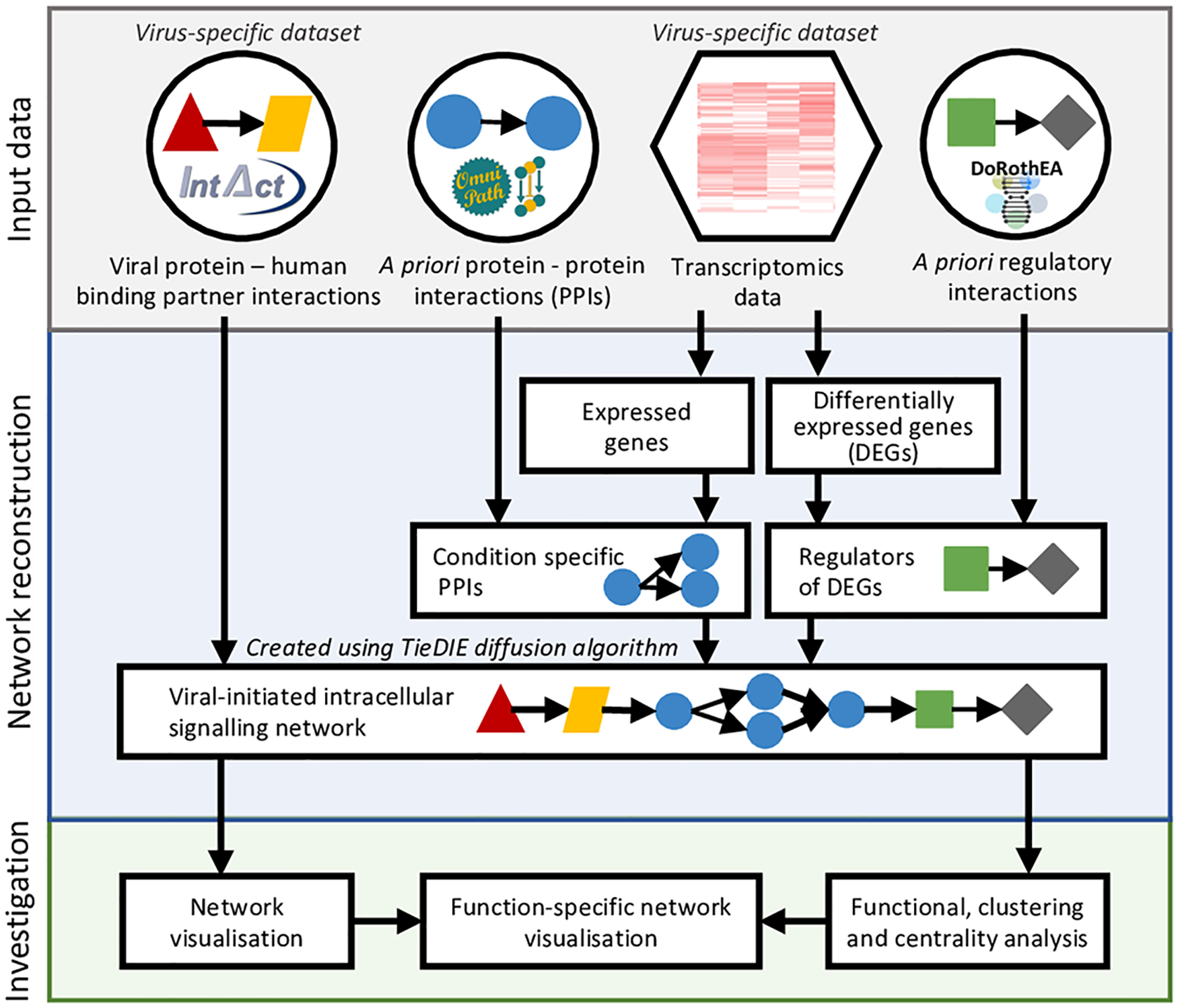
Figure 2: Workflow of the ViralLink tool
For full-sized version, please click on the image.
As a use case of our computational workflows, in a joint project with Simon Carding’s group in the Quadram Institute, we explored how secreted vesicles from gut commensals, such as Bacteroides thetaiotaomicron (Bt), modulate the immune system in a healthy colon and compared to an inflammation on cell type level using single-cell RNAseq data [Figure 3].
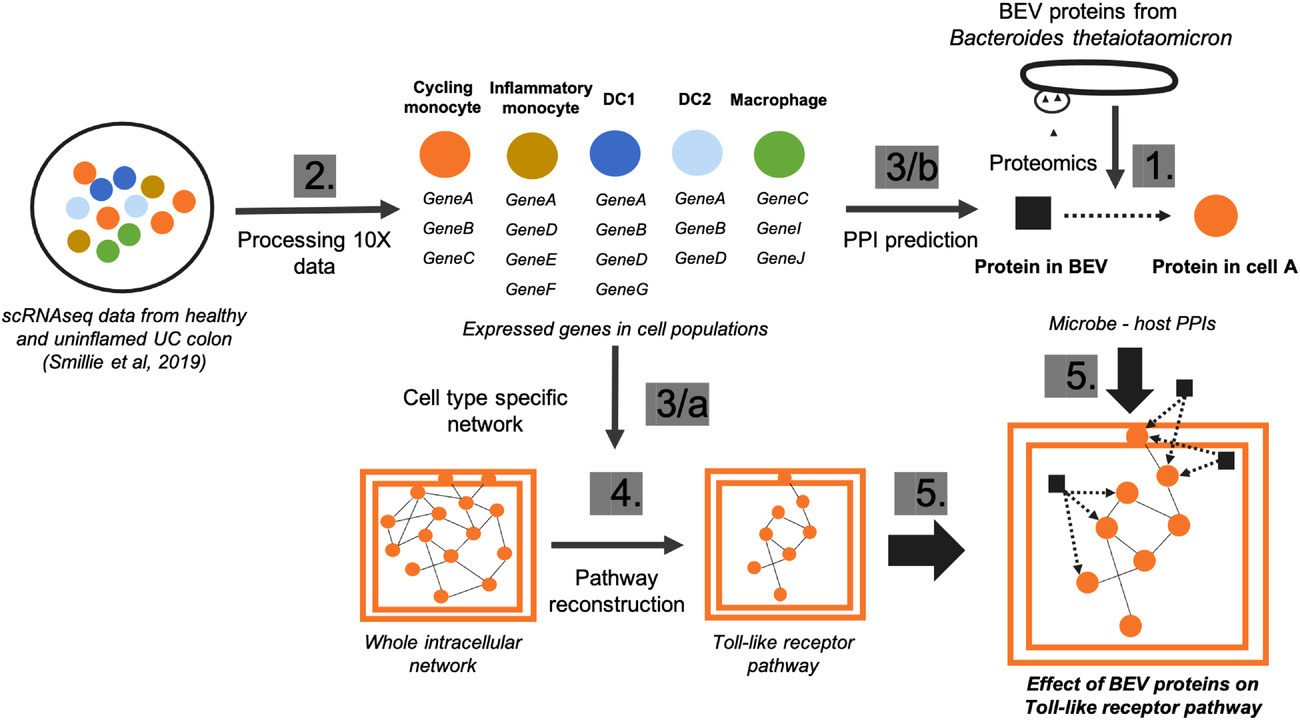
Figure 3: Multi-omics analysis of host-microbe interactions. Numbers indicate the sequence of the main steps: 1, Extraction of bacterial proteins from proteomic dataset; 2, Processing raw single-cell transcriptomics data; 3/a, Creating cell type-specific network using protein-protein interactions from OmniPath; 3/b Predicting protein-protein interactions (PPIs) bacterial and host proteins in each cell type separately using MicrobioLink; 4, Reconstruction of host pathways using Reactome database; 5, Combining cell specific signalling with bacteria targeted human proteins.
For full-sized version, please click on the image.
In summary, our computational workflows provide a detailed insight into the cell-type and condition-specific effect of various microbial communities on host signalling. We plan to extend our pipeline to metabolite-protein interactions, therefore exploring metabolic interaction between microbes and humans.